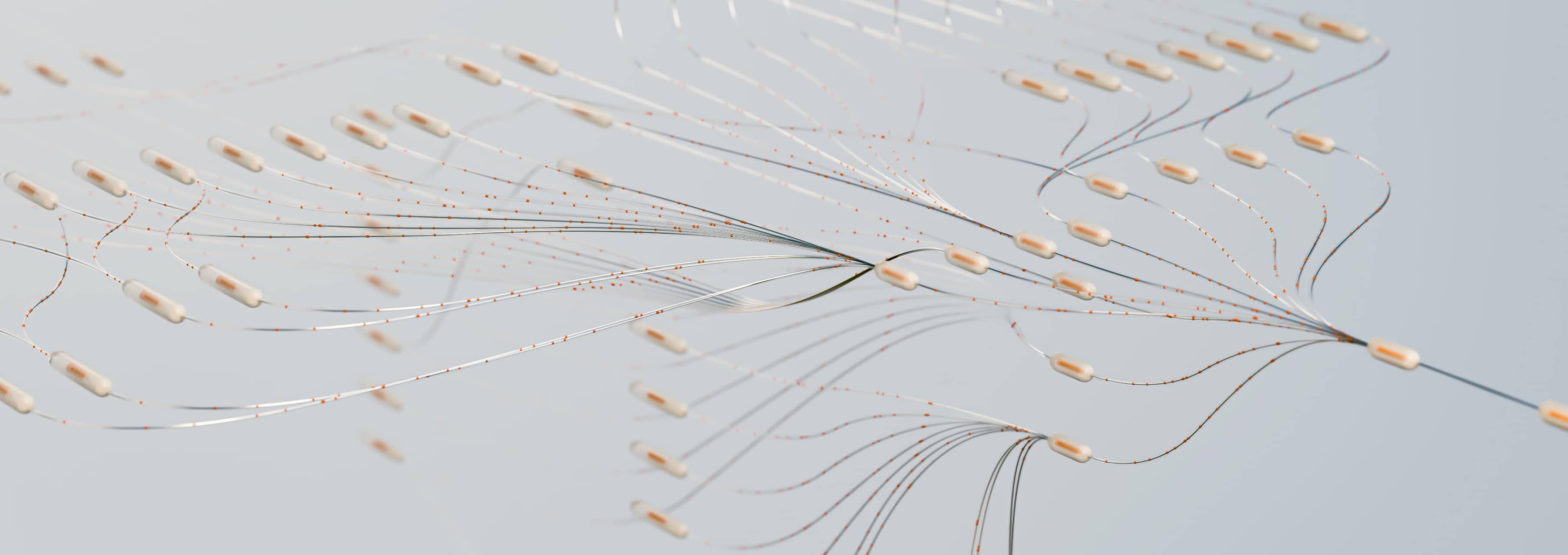
The arrival of Industry 5.0 marks the beginning of a new era in manufacturing that is intelligent, sustainable, and human-centred. As industries worldwide embrace digital transformation, edge computing has emerged as a critical enabler for realising the full potential of Industry 5.0. By bringing data processing and analysis closer to the source, edge computing addresses key challenges around latency, bandwidth, and security—paving the way for revolutionary industrial applications.
The promise of Industry 5.0
By 2025, humans and machines will spend an equal amount of time on current tasks at work.
Industry 5.0 builds upon the digital transformation of Industry 4.0, introducing a more balanced approach that emphasises human-machine collaboration, sustainable practices and resilience. This shift reflects a growing awareness that pure automation isn't always the answer. Industry 5.0 seeks to combine the strengths of human workers with the capabilities of advanced technologies.
Key pillars of Industry 5.0 include:
- Human-centricity: leveraging human creativity and problem-solving in tandem with advanced automation and robotics.
- Sustainability: optimising resource usage and minimising environmental impact through circular economy practices.
- Resilience: building robust, adaptable systems that can withstand disruptions.
- Mass customisation: enabling efficient production of personalised products at scale.
- Cognitive manufacturing: utilising AI and machine learning to enable predictive, self-optimising production systems.
Edge computing provides the foundation to realise these Industry 5.0 objectives by enabling real-time data processing, analytics, and decision-making at the network edge—closer to where data is generated by industrial IoT devices, sensors, and machines. Let’s explore this concept in more detail.
Use cases for Industry 5.0 in industrial applications
Automotive
Allowing customers to fully customise their vehicles, from exterior colours to interior features, using digital configurators. The manufacturing process adapts in real-time to produce these unique vehicles efficiently.
Consumer electronics
Enabling customers to design their own smartphones or laptops with specific components, form factors, and features. AI and robotics work together to assemble these custom devices.
Aerospace assembly
Utilising cobots to assist human workers in precise aircraft assembly tasks. The cobots handle heavy lifting and repetitive tasks, while humans focus on complex decision-making and quality control.
Pharmaceutical production
Employing collaborative robots in clean room environments to work alongside human scientists in drug manufacturing, enhancing precision and reducing contamination risks.
Circular economy in electronics
Implementing AI-driven disassembly lines where robots and humans work together to efficiently recycle and upcycle electronic waste, recovering valuable materials.
Green energy production
Using smart manufacturing techniques and human expertise to produce more efficient solar panels or wind turbines, optimising the use of materials and reducing waste.
Food and beverage industry
Using computer vision and AI for initial quality checks, with human experts making final decisions on complex quality issues. This combination ensures both efficiency and high standards.
Textile manufacturing
Implementing AI-powered defect detection systems that work in tandem with human inspectors to ensure fabric quality, combining machine precision with human judgment.
Smart factories
Using AI and IoT to optimise energy usage in manufacturing facilities in real-time. Human managers oversee the system and make strategic decisions about energy investments and sustainability initiatives.
Utilities
Implementing smart grid technologies that use AI for load balancing and predictive maintenance, with human operators managing complex scenarios and customer interactions.
Edge-enabled Industry 5.0 architecture
The Edge-enabled architecture can be described as a layered system. To better understand it, let’s think of it as a pyramid.
At the most basic level, we have sensors, machines, and devices collecting data. Just above, we have edge nodes and gateways. They process time-sensitive data on the spot, making quick decisions without the delay of sending information to a distant data centre. Then, we have a network layer that connects everything and uses technologies like 5G or industrial Ethernet. Finally, we have the cloud, which covers long-term planning, complex analysis, and decisions that affect the entire operation.
This layered approach allows for the speed and responsiveness of local processing where it matters most, combined with the power and oversight of cloud computing for broader insights. For businesses, this means faster responses to changing conditions, more efficient use of network resources, and the ability to keep sensitive data local when needed.
Edge-enabled Industry 5.0 architecture
Looking ahead, exciting opportunities are emerging at the crossroads of edge computing with other transformative technologies:
- Edge-cloud continuum: seamless integration of edge and cloud resources for optimal workload placement.
- Digital twins: high-fidelity virtual replicas of physical assets and processes enabled by edge data.
- Metaverse for industry: immersive virtual environments for design, training, and remote collaboration.
- Autonomous systems: self-governing industrial systems powered by edge AI and robotics.
Edge AI: bringing intelligence to industrial operation
Edge AI is a cornerstone of Industry 5.0, integrating artificial intelligence and machine learning directly into edge devices and local servers. This allows AI models to run locally, reducing latency and enabling real-time inferencing and decision-making.
By processing data at the source, Edge AI overcomes the limitations of cloud-based AI solutions, particularly in industrial settings where network connectivity may be unreliable or bandwidth-constrained. This approach not only enhances response times but also improves data privacy and security by minimising the need to transmit sensitive information to centralised cloud servers.
- In the context of Industry 5.0, Edge AI facilitates:
-
- Real-time process optimisation: AI models can analyse production data on-the-fly, making immediate adjustments to enhance efficiency and quality.
- Predictive maintenance: By continuously monitoring equipment performance, Edge AI can forecast potential failures before they occur, reducing downtime and maintenance costs.
- Adaptive human-machine interfaces: Edge AI enables more intuitive and responsive interactions between workers and smart machines, supporting the human-centric focus of Industry 5.0.
- Personalised production: AI at the edge can rapidly process customer specifications and adjust manufacturing parameters in real-time, enabling mass customisation.
- Enhanced safety and quality control: Computer vision models running on edge devices can perform instant visual inspections and detect safety hazards.
- Energy efficiency: Edge AI can optimise energy consumption across industrial operations, supporting sustainability goals.
As Edge AI capabilities continue to advance, driven by innovations in hardware accelerators, model compression techniques, and distributed learning algorithms, it will play an increasingly central role in realising the vision of intelligent, adaptive, and human-centric manufacturing that defines Industry 5.0.
Edge computing use cases for Industry 5.0
As industry evolves towards the human-centric approach of Industry 5.0, edge computing will play a crucial role in enabling transformative capabilities:
- Human-machine collaboration. Edge computing facilitates seamless collaboration between human workers and intelligent machines or "cobots". Real-time analytics and adaptive controls enable cobots to work safely alongside people.
- Personalised production. By processing data at the edge, manufacturers can dynamically configure production to meet individual customer needs, enabling mass customisation.
- Predictive maintenance. Edge devices can leverage sensor data and advanced analytics to forecast equipment issues, triggering proactive maintenance and minimising downtime.
- Quality control. Distributed edge-enabled inspection systems can instantly detect defects along the production line, improving quality and reducing waste.
- Process optimisation. Edge computing allows for continuous monitoring and analysis of production data to identify inefficiencies and opportunities for improvement.
- Worker safety. Edge-powered vision systems and wearables can detect unsafe conditions or behaviours in real-time to prevent accidents.
- Energy management. Edge devices can optimise energy consumption across factory equipment and systems based on real-time monitoring and predictive modelling.
By bringing intelligence, autonomy, and adaptability to the industrial edge, edge computing is a foundational technology for realising the human-centric, flexible, and sustainable vision of Industry 5.0.
Challenges and solutions in edge computing adoption for Industry 5.0
While edge computing offers significant benefits, several challenges need to be addressed for successful adoption in Industry 5.0.
Standardisation
Challenge: Ensuring interoperability across diverse edge platforms and devices.
Solution: Industry consortia and standards bodies are developing open protocols and reference architectures for edge computing. Adopting these standards, such as those from the Edge Computing Consortium or the OpenFog Consortium, can enhance interoperability. Additionally, using container technologies and microservices architectures can help create more portable and interoperable edge applications.
Scalability
Challenge: Managing and orchestrating thousands of edge nodes efficiently.
Solution: Implementing robust edge orchestration platforms that support automated deployment, scaling, and management of edge applications. Technologies like Kubernetes adapted for edge environments (e.g., K3s, KubeEdge) can provide scalable container orchestration. Additionally, adopting edge-specific management tools and practices from major cloud providers can facilitate large-scale edge deployments.
Skills gap
Challenge: Training the workforce to develop and maintain edge systems.
Solution: Investing in comprehensive training programs to upskill existing staff in edge computing technologies. Partnering with educational institutions to develop curricula focused on edge computing, IoT, and related technologies. Leveraging managed edge services and vendor support during the initial adoption phase can also help bridge the skills gap while internal expertise is developed.
Business models
Challenge: Defining new value propositions and monetisation strategies for edge services.
Solution: Developing innovative business models that capitalise on the unique capabilities of edge computing, such as:
- Pay-per-use models for edge processing and storage
- Subscription-based edge analytics services
- Outcome-based pricing models tied to specific industrial improvements enabled by edge computing
- Edge-as-a-Service offerings that combine hardware, software, and management
Security
Challenge: Ensuring robust security across distributed edge environments.
Solution: Implementing a comprehensive edge security strategy that includes:
- Hardware-based security features in edge devices
- Zero-trust security models
- End-to-end encryption for data in transit and at rest
- Regular security audits and penetration testing
- Automated security patching and updates for edge nodes
By proactively addressing these challenges with targeted solutions, organisations can smooth the path to edge computing adoption in Industry 5.0, unlocking its full potential to drive innovation, efficiency, and human-centric manufacturing.
Conclusions
Edge computing is a cornerstone technology for implementing the vision of Industry 5.0—enabling intelligent, resilient, and human-centric industrial systems. By bringing compute power closer to data sources, edge computing addresses key challenges around latency, bandwidth, and security while enabling revolutionary applications across manufacturing, healthcare, energy, and beyond.
As we stand on the cusp of this new industrial era, organisations must start building the skills, infrastructure, and partnerships needed to harness the power of edge computing. Those who successfully navigate this transition will be well-positioned to thrive in the intelligent, sustainable industries of tomorrow.
FAQs
Industry 5.0 is the next phase in industrial evolution, focusing on the collaboration between humans and advanced technologies. It aims to combine the high-speed efficiency of Industry 4.0's automation and data exchange with human intelligence, creativity, and problem-solving skills.
Edge computing is a distributed computing paradigm that brings data storage and computation closer to the sources of data. Instead of relying solely on centralised cloud servers, edge computing processes data near the "edge" of the network, where it is generated.