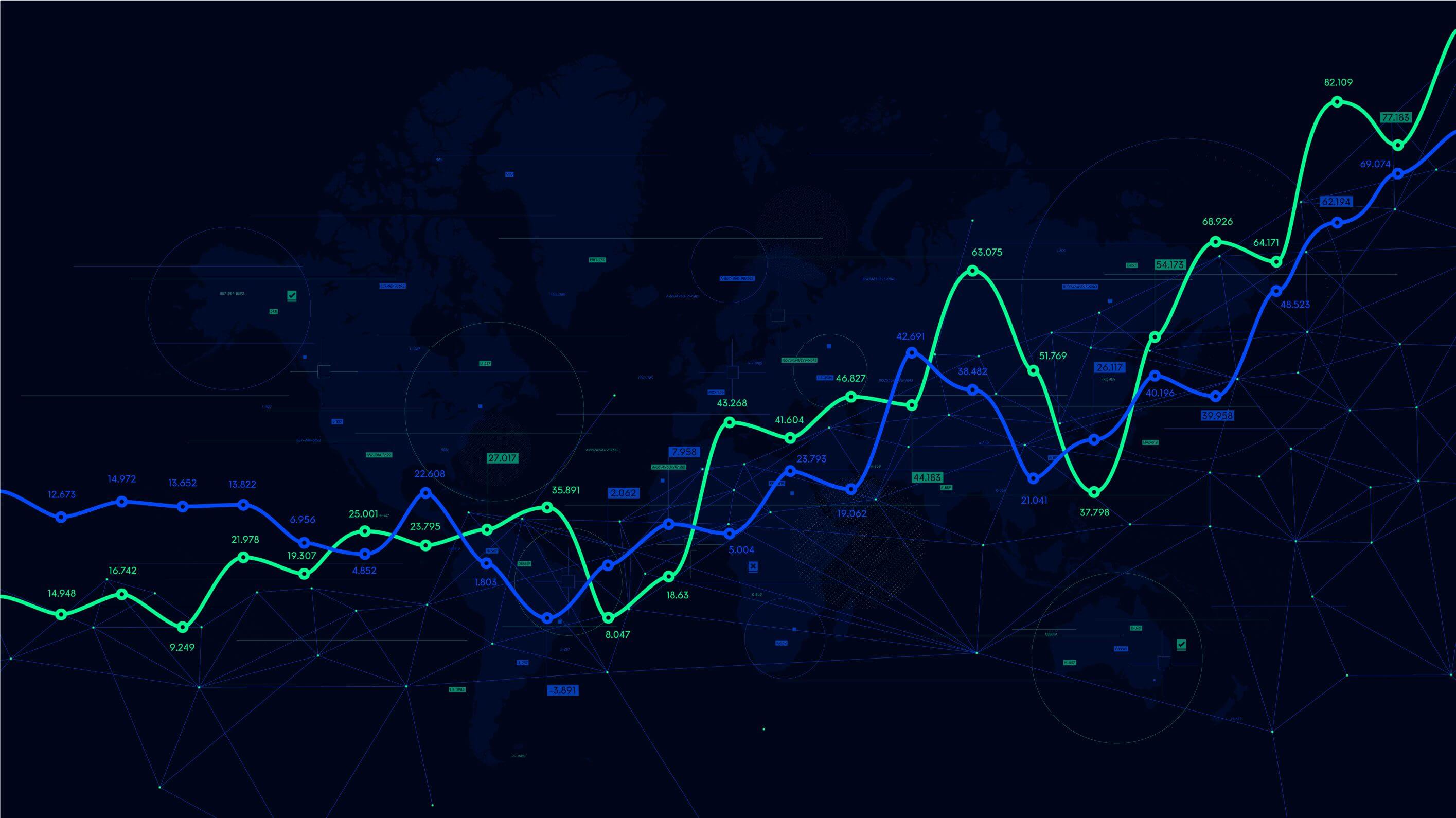
The problem with data analytics
Vast pools of data are one of the signs of our times but turning large amounts of data into meaningful insight has so far remained a challenge. Data science experts commonly spend a large proportion of their time – up to 80% – managing and preparing data. But what if this routine work is automated? What if machine learning algorithms can prepare, analyse and interpret data automatically? Enter augmented analytics.
Understanding augmented analytics
Augmented analytics uses a mix of machine learning and artificial intelligence algorithms to automate the data analysis process. An augmented analytics platform can automatically discover data, prepare data, and analyse data with minimal human intervention.
It is important to distinguish the new wave of augmented analytics from existing systems that aid data analysis. Yes, numerous solutions exist that can “support” data analysis by providing visual aids and by making analytical tasks easier. Instead, augmented analytics automate difficult tasks that ordinarily still require data scientists:
Pattern recognition. Just because data exists does not mean that analysing that data will deliver actionable insights. Pattern recognition involves sorting through batches of data to find the data sets that carry useful information and to eliminate data that is merely noise. Augmented analytics can automatically detect strong data signals.
Insight generation. Significant facts do not automatically equate to insight. For example, knowing that regional sales have increased is useful, but knowing why this occurred can make all the difference. Machine learning algorithms help automate the process of understanding what exactly it is your data is telling you about your organisation.
Augmented analytics takes the legwork out of data manipulation and analysis. Instead, your data scientists can focus on interpreting insights and turning these insights into actions. But how will this benefit enterprises in the real world?
Augmented analytics in practice
As a bleeding-edge technology, the benefits of augmented analytics are still emerging. Nonetheless, the leading vendors in analytics are already offering practical applications that have significant real-world use:
- Data correlation. Instead of manually searching for meaningful correlation, augmented analytics can highlight correlating factors that are significant. Augmented data analytics can also automate the identification of data clusters, broadly speeding up the process of finding significance in data which saves a huge amount of time.
- Natural language queries. Machine learning is useful beyond the analysis of data. Algorithms can also enable data queries, allowing end-users to use natural language to trigger queries. In response, an application can automatically select data, generate code and find data patterns.
- Reduce data bias. Humans are biased when it comes to the process of selecting data for analysis and review. Machines, by definition, are not. Instead, the processing power of a machine allows it to examine all data with no bias with regards to the utility of the data. In turn, your enterprise can draw insight from a wider basis.
Nevertheless, there are still multiple tasks, requiring a deep understanding of the business context, objectives and constraints, which even robust automation methods won't be able to handle effectively. Using augmented data analytics at the beginning of the problem-solving process can be highly recommended, but afterwards, the real work of a data science expert is going to start.
Getting started with augmented analytics
It is important to note that even when augmented with machine learning and AI, data analytics can never improve on poor data. Data that is fundamentally incorrect, collected in a biased manner or otherwise unreliable will not be improved by augmented analytics. Good data sources, through data collection and practising data hygiene, is, therefore, the first step.
Next, enterprises should always involve expert oversight when it comes to data analysis, even if augmented by AI and machine learning. Don’t have in-house data scientists? Consider bringing on board a vendor with data analytics experience.
At ELEKS we know how advanced data analytics can benefit enterprise data insight. Contact us to accelerate your enterprise data analytics.